The Third Workshop for the Interdepartmental Center SmartData@PoliTO will be held on September 24 and 25, 2018 in Turin at the wonderful castle of Valentino, which is the seat of the Architecture Faculty of Politecnico di Torino.
We will meet to present and discuss activities carried over in the center, with invited speakers presenting their activities, and PhD students discussing their ongoing work. A two half-day workshop, in which we will have the chance to meet the External Advisory Board of the center, Prof. Pietro Michiardi (EURECOM), Prof. Sandra di Rocco (KTH), and prof. Alessandro Laio (SISSA). The goal is to share and check the status of the activities, and shape future directions.
Topics:
Cloud Data; Deep learning in healtcare; Machine learning for fracture networks; Cybersecurity; Disease diffusion; Navigation in high dimensional spaces; Document summarization; Graph convolution; Scheduling in Big Data; and more
Location:
Castello del Valentino, salone d’onore
Viale Pier Andrea Mattioli, 39 – 10125 Torino (TO)
Agenda
Monday, September 24th
12:30 – 14:00 – Getting together – Welcome reception with buffet
14:00 – 14:15 Welcome message from Prof. Luca Settineri – Vice Rector of Policy Planning and Implementations
14:15 – 14:45 Presentation of the SmartData@Polito center – Marco Mellia
14:45 – 15:45 Keynote speech 1: Practical and Scalable Probabilistic Machine Learning
– Pietro Michiardi – EAB member
Abstract: In this talk I will first present an overview of the research challenges we address in my group, in the context of probabilistic machine learning. With this context in mind, I will delve into the details of recent approaches to achieve a practical and scalable way to train deep probabilistic models built on the concept of Gaussian Processes. To this end, I will first briefly introduce what Gaussian Process inference is, then I will move on to describe a model approximation technique, based on random Fourier features, and approximate variational inference to train the resulting model. The talk will conclude with a distributed systems flavour, covering methods and systems to train large scale machine learning models, including data and model parallelism approaches.
15:45 – 16:15 Technical presentation A: Deep learning applications in healthcare – Lia Morra
Abstract: Machine learning and, more recently, deep learning are powerful tools that will revolutionize the way healthcare is implemented and delivered to patients. The field of radiology has pioneered this revolution, thanks to the early availability of relatively large retrospective archives in digital format. Nonetheless, learning from health data poses many challenges due to the lack of quality annotated data, high class imbalances and multiple sources of biases. In this talk, I will illustrate how these challenges can be addressed in practice, e.g. by exploiting techniques such as transfer learning, and provide some illustrative examples in early cancer detection and diagnosis. I will also discuss about specific challenges related to technology transfer from the lab to the clinic (considering, e.g., regulation, quality assessment and data management issues), drawing from hands-on experience in both industry and academia, and how these stringent requirements have shaped the research field in the past years.
16:15 – 16:45 Technical presentation B: Machine Learning for Fluxes Regression in Discrete Fracture Networks – Francesco Della Santa
Abstract: Flow and transport characterisation through fractured media in the subsurface is a crucial theme in many applications concerning civil, environmental and industrial engineering, e.g. in oil and gas extraction or in avoidance of drinking water pollution due to industrial waste. Therefore all of these applications require models that can simulate flow through networks of subsurface fractures as best as possible.Unfortunately the knowledge of rules that regulate the topology of these sparse systems of fracture networks is quite unknown since exact positions of fractures (located hundreds of meters underground) usually and very often can’t be determined; then geologically-realistic statistical representations of fracture networks (the so-called Discrete Fracture Networks – DFNs) are used to simulate flow and transport through fractured media. Due to probabilistic nature of DFNs, flow and transport characterisation in a real fractured medium usually require a statistical analysis of thousands of DFNs simulations; therefore, in order to speed up simulations processes, machine leaning methods could introduce a new interesting approach. In this talk, some Neural Networks (NNs) applications for fluxes regression problems in a fixed-geometry DFN will be described. These kind of problems perform vector-valued regression, through NNs, of m out-flow fluxes for a given DFN, varying with transmissivity values of n fixed fractures among the N total ones. Therefore, varying with the number n of fractures considered, but also with NNs’ regularisation and architecture, behaviour of regression quality have been studied and will be illustrated.
16:45 – 17:00 Coffee Break
17:00 – 17:45 Poster session (PhD Students)
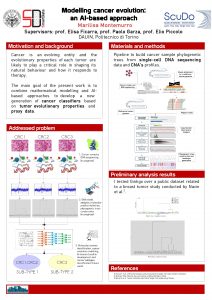
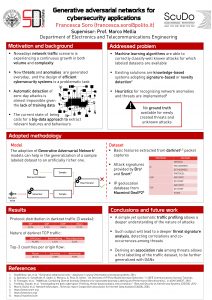

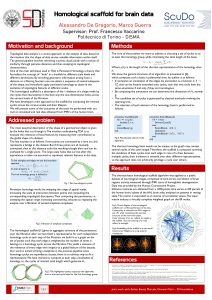





Tuesday, September 25th
09:00 – 09:45 Keynote speech 2: Variety sampling – Sandra Di Rocco – EAB member
Point data-clouds are the objects of exciting research and advances in modern analysis of data. I will present some theoretical advances and some numerical algorithms connected to sampling algebraic models.
09:45 – 10:15 Technical presentation C: Automatic Longitudinal Exploration of Network Traffic Analysis and applications to cybersecurity – Andrea Morichetta
Abstract: In this work, we present LENTA (Longitudinal Exploration for Network Traffic Analysis), a system that sup- ports the network analysts to easily identify traffic generated by services and applications running on the web, being them benign or possibly malicious. First, LENTA simplifies analysts’ job by letting them observe few hundreds of clusters instead of the original hundred thousands of single URLs. Second, it implements a self-learning methodology, where a semi-supervised approach lets the system grow its knowledge, which is used in turn to automatically associate traffic to previously observed services and identify new traffic generated by possibly suspicious applications. This lets the analysts easily observe changes in the traffic, like the birth of new services, or unexpected activities. We follow a data driven approach, running LENTA on real data. Traffic is analyzed in batches of 24-hour worth of traffic. We show that LENTA allows the analyst to easily understand which services are running on their network, highlights malicious traffic and changes over time, greatly simplifying the view and understanding of the traffic.
10:15 – 10:45 Technical presentation D – Computational framework for inference in epidemic processes on livestock movement networks – Indaco Biazzo
Abstract: Zoonotic diseases have a huge impact in the cattle industry productivity and represent a threat to human health. Studying livestock movements is of primary importance to obtain an accurate knowledge of the existence of direct and indirect contagion channels. Just in Italy, the network of cattle movements include each year the transport of more than 7 millions animals between hundred of thousand of farms and other structures. Recent studies show a complicated interplay between contagions through direct cattle-cattle contact and other indirect channels (e.g. contact with wild animals). Having an accurate model of the contagion channels and potential causal relations is of primary importance in livestock surveillance. Models for such a complex scenario usually involve a large number of parameters. Common methods for risk assessment analysis require to perform an enormous number of Monte-Carlo simulations to sample the space of initial conditions and model parameters that is compatible with observations. In the talk, a computational statistics framework based on Belief Propagation is presented. The framework allows us to simultaneously infer the huge number of parameters of the contagion model as well as the actual infection tree, having partial observation data of the contagion process.
10:45 – 11:00 Coffee Break
11:00 – 11:45 Keynote speech 3 – Synthetic maps for navigating high-dimensional data spaces – Alessandro Laio – EAB member
Abstract: Data analysis in high-dimensional spaces aims at obtaining a synthetic description of a data set, revealing its main structure and its salient features. We will describe an approach for charting data spaces, providing a topography of the probability distribution from which the data are harvested. This topography includes information on the number and the height of the probability peaks, the depth of the “valleys” separating them, the relative location of the peaks and their hierarchical organization. The topography is reconstructed by using an unsupervised variant of Density Peak clustering exploiting a non-parametric density estimator, which automatically measures the density in the manifold containing the data. Importantly, the density estimator provides an estimate of the error. This is a key feature, which allows distinguishing genuine probability peaks from density fluctuations due to finite sampling.
11:45 – 12:15 Technical presentation E: Extractive multilingual document sumarization – Luca Cagliero
Extractive multi-document summarization addresses the selection of a compact subset of highly informative sentences, i.e., the summary, from a collection of textual documents. To perform sentence selection, two parallel strategies have been proposed: (a) apply general-purpose techniques relying on data mining or information retrieval techniques, and/or (b) perform advanced linguistic analysis relying on semantics-based models (e.g., ontologies) to capture the actual sentence meaning. Since there is an increasing need for processing documents written in different languages, the attention of the research community has recently focused on summarizers based on strategy (a). In this presentation, we overview the main challenges related to summarization of documents written in different languages and we present an itemset-based summarization approach that makes minimal use of language-dependent analyses while considering correlations among multiple highly relevant terms that are neglected by previous approaches.
12:15 – 12:45 Technical presentation F: Learning Localized Generative Models for 3D Point Clouds via Graph Convolution – Giulia Fracastoro
Abstract: Point clouds are an important type of geometric data generated by 3D acquisition devices, and have widespread use in computer graphics and vision. However, learning representations for point clouds is particularly challenging due to their nature as being an unordered collection of points irregularly distributed in 3D space. Graph convolution, a generalization of the convolution operation for data defined over graphs, has been recently shown to be very successful at extracting localized features from point clouds in supervised or semi-supervised tasks such as classification or segmentation. In this presentation, we focus on the unsupervised problem of a generative model exploiting graph convolution. We present a novel architecture that can generate localized features that approximate graph embeddings of the output geometry.
14:00 – 15:30 SmartData@PoliTO Board meeting with EAB members