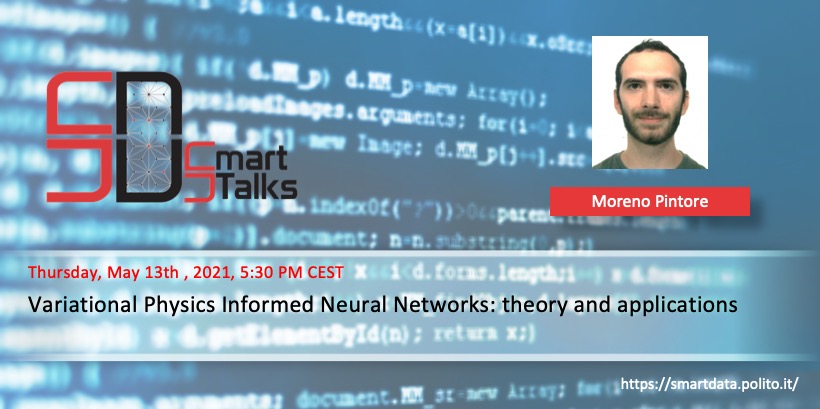
Presenter: Moreno Pintore
Thursday, May 13th, 2021 17:30
Location: Microsoft Teams – click here to join
Moreno Pintore: Variational Physics Informed Neural Networks: theory and applications.
Most of the physical phenomena that are related to engineering applications are governed by partial differential equations (PDEs). Since such equations are often too complex to be analytically solved, it is important to be able to approximate their solutions by means of accurate numerical solvers. One of the most known and used numerical methods is the finite element method. In this talk, a recently developed approach that combines the finite element method with deep neural networks is described and analyzed. It is named Variational Physics Informed Neural Network because the solution is approximated employing a neural network trained using the physics of the problem with a variational approach. This new technique can be used to solve several PDEs with only slight changes in the implementation and it can compute accurate solutions as high order classical techniques. During the talk, the abstract formulation, a novel a priori error estimate and possible future applications will be described.
Biography: I studied Mathematical engineering at Politecnico of Torino. During the bachelor’s thesis I faced geometrical problems associated to scientific computing while, in the master’s one, I used the reduced basis method, the spectral element, the continuation and the deflation ones to numerically compute bifurcation diagrams.