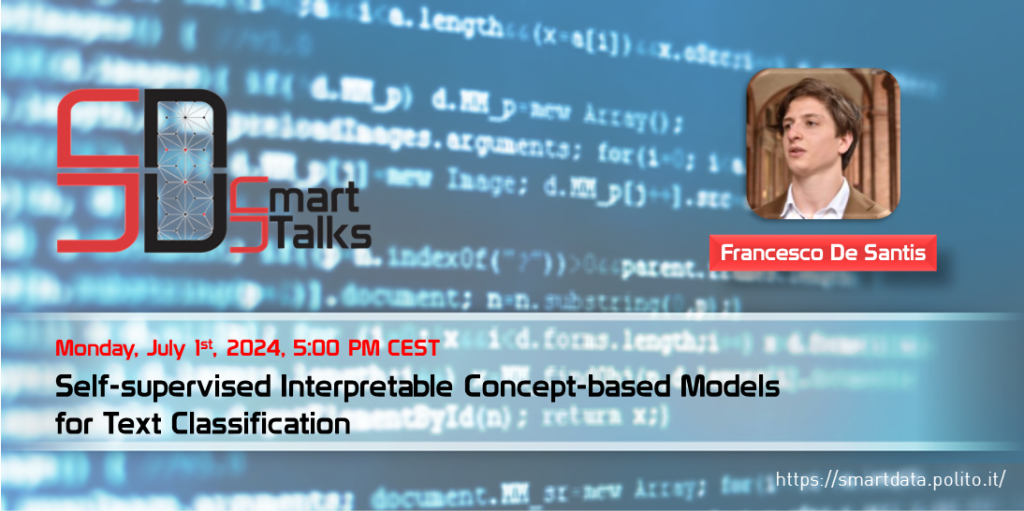
Presenter: Francesco De Santis
Monday, July 1st, 2024 5:00 PM
Location: Sala Piccola Polito@Covivio, Corso Ferrucci 112
ABSTRACT
Despite their success, Large-Language Models (LLMs) still face criticism as their lack of interpretability limits their controllability and reliability. Traditional post-hoc interpretation methods, based on attention and gradient-based analysis, offer limited insight into the model’s decision-making processes.
In the image field, concept-based models have emerged as explainable-by-design architectures, employing human-interpretable features as intermediate representations. However, these methods have not been yet adapted to textual data, mainly because they require expensive concept annotations, which are impractical for real-world text data. In this study we address this challenge by proposing a self-supervised Interpretable Concept Embedding Model (ICEM). We leverage the generalization abilities of LLMs to predict the concepts labels in a selfsupervised way, while we deliver the final predictions with an interpretable function.
BIOGRAPHY
Francesco De Santis obtained his Master’s degree in Data Science for Engineering in 2022 (Politecnico di Torino). Now he is a Phd student in Computer and Control Engineering at Politecnico di Torino and a member of the SmartData research group. His main research interest is in LLMs interpretability.