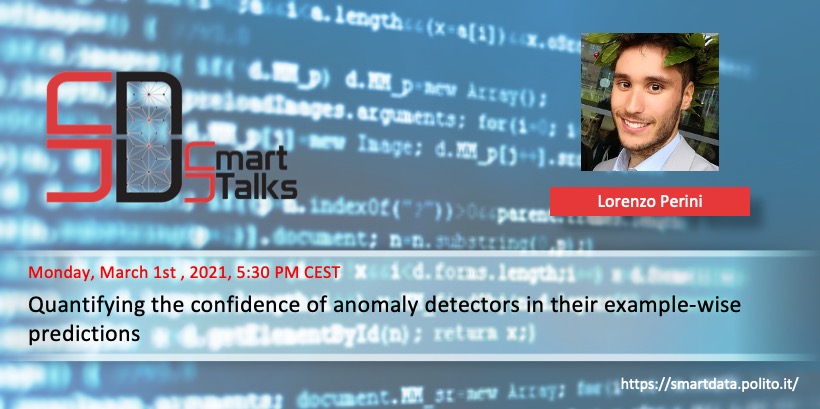
Presenter: Lorenzo Perini
Monday, March 1st, 2021 17:30
Location: Microsoft Teams – click here to join
Lorenzo Perini: Quantifying the confidence of anomaly detectors in their example-wise predictions
Anomaly detection focuses on identifying examples in the data that somehow deviate from what is expected or typical. Algorithms for this task usually assign a score to each example that represents how anomalous the example is. Then, a threshold on the scores turns them into concrete predictions. However, each algorithm uses a different approach to assign the scores, which makes them difficult to interpret and can quickly erode a user’s trust in the predictions. To overcome this limitation, we introduce an approach for assessing the reliability of any anomaly detector’s example-wise predictions. To do so, we propose a Bayesian approach for converting anomaly scores to probability estimates. This enables the anomaly detector to assign a confidence score to each prediction which captures its uncertainty in that prediction. Specifically, the confidence measures the probability that any example’s predicted class would change if a different training set was observed. We theoretically analyze the convergence behaviour of our confidence estimate. Empirically, we demonstrate the effectiveness of the framework in quantifying a detector’s confidence in its predictions on a large benchmark of datasets.
Biography: Lorenzo Perini is a second-year Ph.D. student in the Machine Learning Group of the Department of Computer Science at KU Leuven (Leuven, Belgium), under the supervision of Professor Jesse Davis. After he obtained a Bachelor Degree of Mathematics in July 2017 with a thesis in Probability, he studied at Politecnico di Torino for two years and earned the Master Degree of Mathematical Engineering in July 2019. He specialized in Machine Learning with an applied thesis about predictive maintenance and anomaly detection, which was done in collaboration with the company Tierra S.p.A. His current research interests are PU Learning, Active Learning, and Transfer Learning with applications to the field of Anomaly Detection. Moreover, he mainly focuses on probabilistic approaches and is now working on measuring and exploiting the models’ uncertainty to improve their robustness.